概述
文章目录
- 1. 早期停止法
- 1.1 Python 实现
- 参考文献
相关文章:
机器学习 | 目录
机器学习 | 梯度下降原理及Python实现
1. 早期停止法
对于梯度下降这一类迭代学习的算法,还有一个与众不同的正则化方法,就是在验证误差达到最小值时停止训练,该方法叫作早期停止法
。下图展现了一个用批量梯度下降训练的复杂模型(高阶多项式回归模型)。经过一轮一轮的训练,算法不断地学习,训练集上的预测误差(RMSE)自然不断下降,同样其在验证集上的预测误差也随之下降。但是,一段时间之后,验证误差停止下降反而开始上升。这说明模型开始过度拟合训练数据。通过早期停止法,一旦验证误差达到最小值就立刻停止训练。这是一个非常简单而有效的正则化技巧。[1]
对随机梯度下降和小批量梯度下降来说,曲线没有那么平滑,所以很难知道是否已经达到最小值。一种解决办法是等验证误差超过最小值一段时间之后再停止(这时你可以确信模型不会变得更好了),然后将模型参数回滚到验证误差最小时的位置。
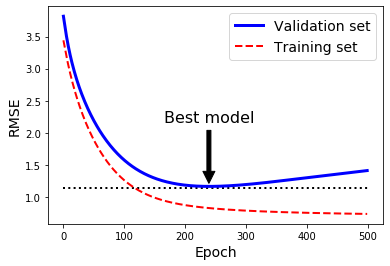
1.1 Python 实现
import numpy as np
import matplotlib.pyplot as plt
np.random.seed(42)
m = 100
X = 6 * np.random.rand(m, 1) - 3
y = 2 + X + 0.5 * X**2 + np.random.randn(m, 1)
from sklearn.model_selection import train_test_split
X_train, X_val, y_train, y_val = train_test_split(X[:50], y[:50].ravel(), test_size=0.5, random_state=10)
from sklearn.pipeline import Pipeline
from sklearn.preprocessing import PolynomialFeatures, StandardScaler
poly_scaler = Pipeline([
("poly_features", PolynomialFeatures(degree=90, include_bias=False)),
("std_scaler", StandardScaler()),
])
X_train_poly_scaled = poly_scaler.fit_transform(X_train)
X_val_poly_scaled = poly_scaler.transform(X_val)
from sklearn.linear_model import SGDRegressor
sgd_reg = SGDRegressor(max_iter=1,
tol=-np.infty,
penalty=None,
eta0=0.0005,
warm_start=True, # 当 warm_start=True 时,调用 fit() 方法,会从停下的地方继续
# 开始训练,而不会重新开始
learning_rate="constant",
random_state=42)
from sklearn.metrics import mean_squared_error
n_epochs = 500
train_errors, val_errors = [], []
for epoch in range(n_epochs):
sgd_reg.fit(X_train_poly_scaled, y_train)
y_train_predict = sgd_reg.predict(X_train_poly_scaled)
y_val_predict = sgd_reg.predict(X_val_poly_scaled)
train_errors.append(mean_squared_error(y_train, y_train_predict))
val_errors.append(mean_squared_error(y_val, y_val_predict))
best_epoch = np.argmin(val_errors)
best_val_rmse = np.sqrt(val_errors[best_epoch])
# 画箭头
plt.annotate('Best model',
xy=(best_epoch, best_val_rmse),
xytext=(best_epoch, best_val_rmse + 1),
ha="center",
arrowprops=dict(facecolor='black', shrink=0.05),
fontsize=16,
)
best_val_rmse -= 0.03 # just to make the graph look better
plt.plot([0, n_epochs], [best_val_rmse, best_val_rmse], "k:", linewidth=2)
plt.plot(np.sqrt(val_errors), "b-", linewidth=3, label="Validation set")
plt.plot(np.sqrt(train_errors), "r--", linewidth=2, label="Training set")
plt.legend(loc="upper right", fontsize=14)
plt.xlabel("Epoch", fontsize=14)
plt.ylabel("RMSE", fontsize=14)
plt.show()
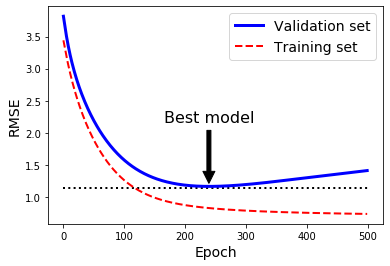
参考文献
[1] Aurelien Geron, 王静源, 贾玮, 边蕤, 邱俊涛. 机器学习实战:基于 Scikit-Learn 和 TensorFlow[M]. 北京: 机械工业出版社, 2018: 125-126.
最后
以上就是瘦瘦电源为你收集整理的机器学习 | 早期停止法原理及Python实现1. 早期停止法参考文献的全部内容,希望文章能够帮你解决机器学习 | 早期停止法原理及Python实现1. 早期停止法参考文献所遇到的程序开发问题。
如果觉得靠谱客网站的内容还不错,欢迎将靠谱客网站推荐给程序员好友。
本图文内容来源于网友提供,作为学习参考使用,或来自网络收集整理,版权属于原作者所有。
发表评论 取消回复