概述
1.1
deepnet = alexnet %利用库内pretrained alexnet
img = imread('filename') %使用变量存储图片
imshow(img) %展示图片
classify(deepnet,img) %使用alexnet来分类当前的图片
1.2
ly=deepnet.Layers %用ly变量存储deepnet的layers信息,如下图
inlayer = ly(1) %提取输入层的信息
insz = inlayer.InputSize %提取输入层的维度
outlayer=ly(end) %提取输出层的信息
categorynames = outlayer.ClassNames %提取输出层的分类名
1.3 对每个类别的输出概率
[pred,scrs] = classify(net,img) %获取每个标签的概率scrs
1.4 创造一个datastore
将图片存在硬盘中即可,使用datastore来索引图片,还可以不必考虑内存使用
imds = imageDatastore('file*.jpg') %创建一个datastore *号代表任意值
fname = imds.Files %提取所有文件的名字
[pred,scores] = classify(deepnet,imds) %将ds的图片都送入进行分类,scores = 文件数*预测单元数
[C,labels]=max(scores,[],2) %C为预测的最大值,labels 为预测的种类标签 长度= 文件数*1
bar(C)
1.5
用自己的文件夹把不同的花进行分类
利用
flwrds= imageDatastore('Flowers','IncludeSubfolders',true);
‘includeSubfolders’可以将子文件夹也包括进去;
2. 迁移学习
迁移学习中我们需要的有三件事。1.选取一个pretrained神经网络 2.所需的数据集 3.超参数的设置
利用文件夹的名字对datastore里的数据进行标记。
load pathToImages
将数据集进行分块
[ds1,ds2]=splitEachLabels(ds,p or n,'randomlize');
防止数据偏斜,将ds的数据随机分为两个部分,p值决定ds1中数据量的占比,n值可以决定ds1中数据量
对预训练的神经网络进行修改
例中我们要识别12种花,我们要将第23层有1000个单元的神经层替换成12个单元的全连接层。再将最后一层1000个类别的输出层换成我们的类别输出层(无需加上类型?);
Layer(23) = fullyConnectedLayer(12)
layers(25) = classificationLayer
修改超参数的设置
opts = trainingOptions('sgdm') %显示随机梯度下降的默认options
opts = trainingOptions('sgdm','name',value) %name中填入对应想修改的参数名
迁移学习总览
Transfer Learning Example Script
trainflowers.mlx
in the course example files. You can download the course example files from the help menu in the top-right corner. Note that this example can take some time to run if you run it on a computer that does not have a GPU.
Get training images
flower_ds = imageDatastore('Flowers','IncludeSubfolders',true,'LabelSource','foldernames'); [trainImgs,testImgs] = splitEachLabel(flower_ds,0.6); numClasses = numel(categories(flower_ds.Labels));
Create a network by modifying AlexNet
net = alexnet; layers = net.Layers; layers(end-2) = fullyConnectedLayer(numClasses); layers(end) = classificationLayer;
Set training algorithm options
options = trainingOptions('sgdm','InitialLearnRate', 0.001);
Perform training
[flowernet,info] = trainNetwork(trainImgs, layers, options);
%可使用 plot(info.TrainingLoss) 绘图loss的变化
Use trained network to classify test images
testpreds = classify(flowernet,testImgs)
correct = nn(AuctualLabels == testpreds) %输出一个分类正确与否的向量
Performance by class
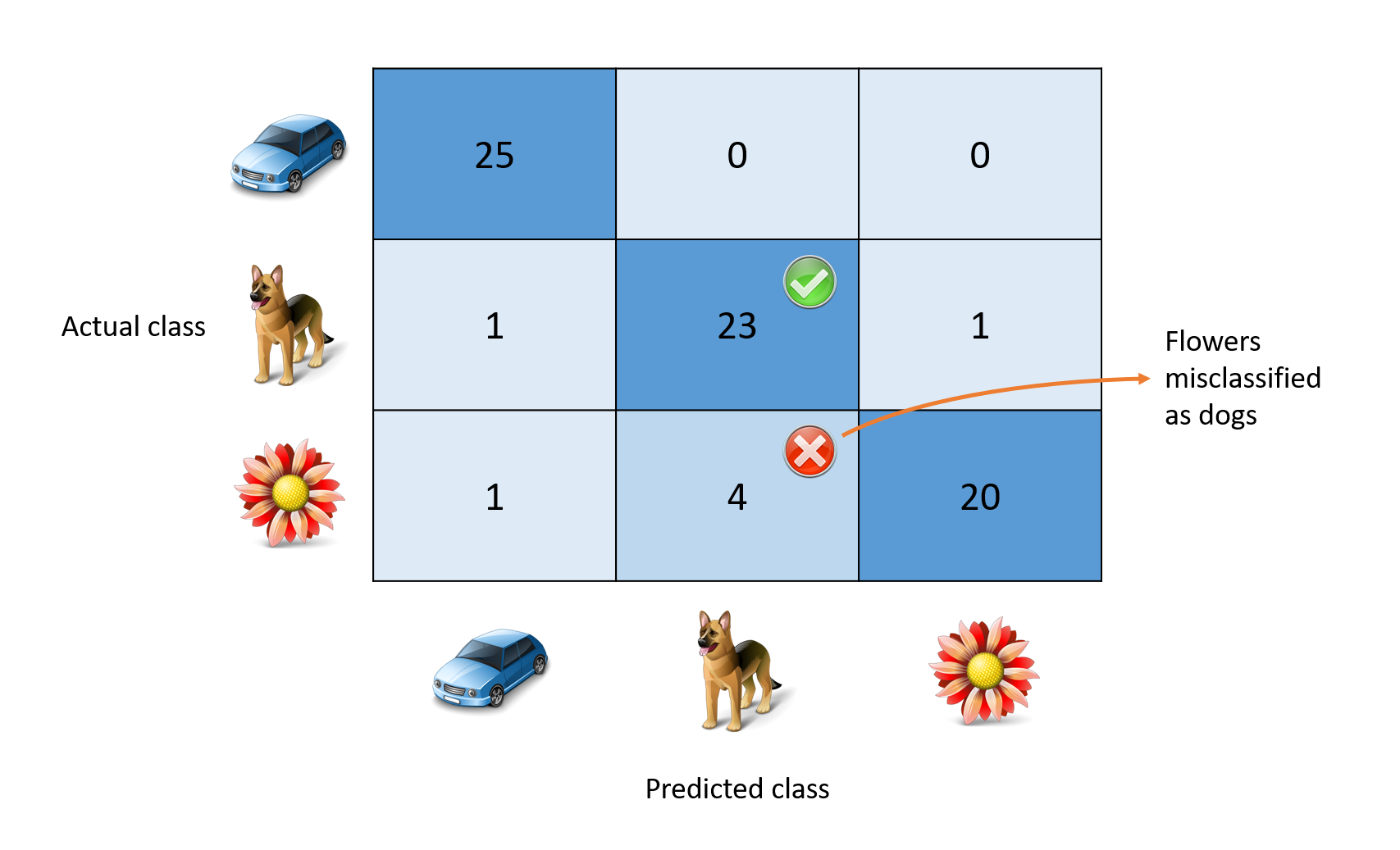
loss与accuracy 给予了全面的关于神经网络表现的信息,但是不能直观的看出那些数据的分类情况
这时候我们使用分类混淆矩阵(confusion matrix)来帮助我们直观的看见哪些数据被误分类
[flwrconf,flwrnames]=confusionmat(testImgs.Labels,flwrPreds)
还是不够直观,我们使用heatmap(xlabels,ylabels,confusionmat)来构建直观的图像
对输入图像进行处理
图像切割
减少图片像素
为图像增加两个通道
wormds = imageDatastore(pathToImages,'ReadFcn',@wormread)
使用DataStore时使用@myfun
fuction myfun
....
end
来自定义读取文件时的操作
img = read(wormds); %读取图片时的操作
通过对图片的修改来增强自己的图片集
最后
以上就是花痴小霸王为你收集整理的Deep Learning Onramp 学习笔记Transfer Learning Example ScriptPerformance by class的全部内容,希望文章能够帮你解决Deep Learning Onramp 学习笔记Transfer Learning Example ScriptPerformance by class所遇到的程序开发问题。
如果觉得靠谱客网站的内容还不错,欢迎将靠谱客网站推荐给程序员好友。
发表评论 取消回复