概述
原文链接:https://dreamhomes.github.io/posts/202101251633.html
文章链接:https://arxiv.org/abs/2101.04977
源码链接:未公布
TL;DR
针对分布式系统服务的异常检测问题,文中提出了一种多模态的异常检测模型,联合了 trace 和 log 数据的特征表示来共同判定异常;对于异常检测任务将其形式化表示成 NTP next template prediction,同时适用于log和trace的异常检测;在实验中论文验证了融合多模态数据比单模态数据异常检测的效果更好。
Problem Statement
Logs
日志可以表示为一种非结构化的时序文本数据,由于log数据中包含很多噪声,因此采用tokenized字典
D
l
o
g
s
_
w
o
r
d
s
D_{logs_words}
Dlogs_words 来表示日志模板,对于不同的log token 使用
<
S
P
E
C
L
O
G
>
<SPECLOG>
<SPECLOG> 进行填充以保证日志具有相同的长度;日志模板可以表示为:
L
i
=
{
W
0
i
,
W
1
i
,
…
,
W
t
i
}
L_{i}=left{W_{0}^{i}, W_{1}^{i}, ldots, W_{t}^{i}right}
Li={W0i,W1i,…,Wti}
其中每个
W
t
W_t
Wt 是对应的索引
t
t
t 从日志中提取的字段
t
∈
D
l
o
g
s
_
w
o
r
d
_
i
n
d
e
c
i
e
s
tin D_{logs_word_indecies}
t∈Dlogs_word_indecies;
Traces
Trace 是对于用户请求记录的一系列执行路径。一个 trace 由多个 spans/events 组成,每个 span 包含请求的一些信息,例如 (start time, end time, service name, HTTP path),因此一个 Trace 可以表示为
T
i
=
{
S
0
i
,
S
1
i
,
…
,
S
m
i
}
T_{i}=left{S_{0}^{i}, S_{1}^{i}, ldots, S_{m}^{i}right}
Ti={S0i,S1i,…,Smi}
注:每个span 包含两种类型的调用: HTTP或者RPC;HTTP一般表示为 path, scheme, method. RPC 调用表示为执行的 functions;
由于Trace数据中也包含噪声,所有文中使用类似日志模板提取的方法来提取 Trace 中的 template spans,可以形式化的表示为
T
i
=
{
S
t
0
i
,
S
t
1
i
,
…
,
S
t
k
i
}
T_{i}=left{St_{0}^{i}, St_{1}^{i}, ldots, St_{k}^{i}right}
Ti={St0i,St1i,…,Stki}
其中每个
S
t
k
St_k
Stk 是对于索引
k
∈
D
t
e
m
p
l
a
t
e
i
n
d
e
c
i
e
s
k in D_{template~indecies}
k∈Dtemplate indecies 提取的模板;
作者观察到Trace 中的每个函数调用都是一个字符序列,因此可以使用定义的字典
D
s
p
a
n
w
o
r
d
s
D_{span~ words}
Dspan words 来表示;每个 span 可以表示为
S
t
j
=
{
W
0
i
,
W
1
j
,
…
,
W
q
j
}
S t_{j}=left{W_{0}^{i}, W_{1}^{j}, ldots, W_{q}^{j}right}
Stj={W0i,W1j,…,Wqj}
每个 span 中包含的关键字段不同,所以文中使用
<
S
P
E
C
S
P
A
N
>
<SPECSPAN>
<SPECSPAN> 来填充缺失的字段。
NTP
基于上述的 trace 和 log 的序列化表示,log 和 trace 异常检测问题的可以形式化地表示为
P
(
A
T
w
i
n
:
T
)
=
∏
t
=
T
w
i
n
T
P
(
A
t
∣
A
<
t
)
Pleft(A_{T_{w i n}: T}right)=prod_{t=T_{w i n}}^{T} Pleft(A_{t} mid A_{<t}right)
P(ATwin:T)=t=Twin∏TP(At∣A<t)
其中 A < t A_{<t} A<t 表历史数据中的 trace 和 log 模板, w i n win win 代表历史时间窗口;因此异常检测的任务就都可以表示为 NTP (next template prediction);
Model / Algorithm
论文中模型的主要思路是根据单模态异常检测的模型进行扩充,同时输入 logs 序列和 traces 序列来判定异常,简单看下单模态的检测模型;
Single-modal
单模态的模型以
D
l
o
g
s
_
w
o
r
d
s
D_{logs_words}
Dlogs_words 或者
D
s
p
a
n
_
w
o
r
d
s
D_{span_words}
Dspan_words 作为输入,以固定长度的随机向量来初始化 words,Template embedding layer 以words 来生成对应的模板序列,然后以这些序列输入 LSTM 来表示序列间的依赖关系
f
(
x
)
f(x)
f(x),输出为预测模板与真实模板间的softmax概率,计算公式如下:
P
(
f
(
x
)
)
=
e
f
(
x
)
∑
i
=
1
A
e
f
i
(
x
)
P(f(x))=frac{e^{f(x)}}{sum_{i=1}^{A} e^{f_{i}(x)}}
P(f(x))=∑i=1Aefi(x)ef(x)
Multi-modal
基于以上单模态模型,为了融合 log 和 trace 两种数据源,论文改进的多模态模型如下所示:
整体架构类似,改进点在于融合了两种数据源输入然后进行特征融合,模型的损失函数考虑了log 和 trace 的联合损失:
L
(
(
s
,
l
)
,
f
(
x
,
y
)
)
=
L
(
f
(
x
)
,
s
)
+
L
(
f
(
y
)
,
l
)
L((s, l), f(x, y))=L(f(x), s)+L(f(y), l)
L((s,l),f(x,y))=L(f(x),s)+L(f(y),l)
其中
L
L
L 表示分类交叉熵损失函数,
s
,
l
s,l
s,l 表示真实的模板;
Anomaly Detection
对于 log 的异常检测,如果预测的模板在 t o p _ k _ l o g s top_k_logs top_k_logs 之内则为正常;对于 trace 异常检测,如果预测的span 在 t o p _ k _ s p a n s top_k_spans top_k_spans 之内则为正常,否则为异常span,当异常span的比例超过定义的异常比例时trace才是异常的;
Experiments
文章在一个开源的数据集上进行测试;数据源 https://github.com/snedelkoski/multi-source-observability-dataset ,实验结果如下,仅和单模态模型进行对比;
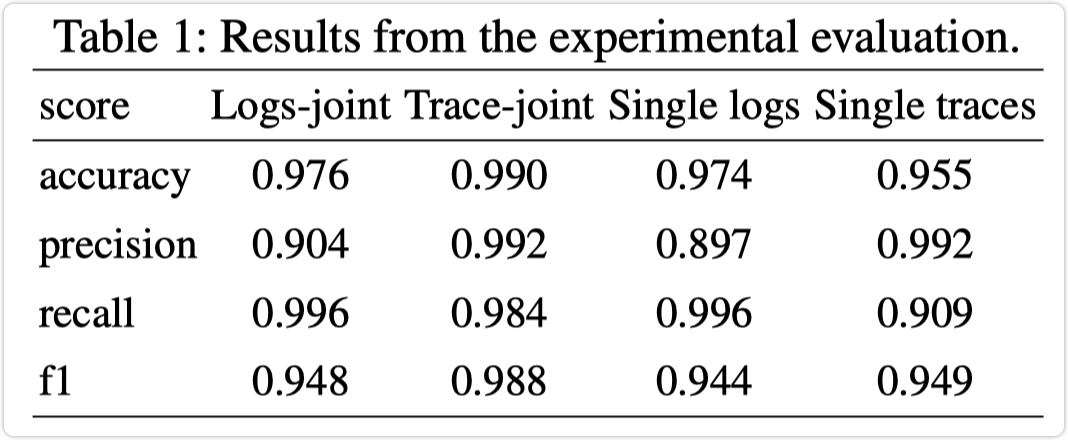
联系作者

最后
以上就是顺心樱桃为你收集整理的【2021】Multi-Source Anomaly Detection in Distributed IT Systems的全部内容,希望文章能够帮你解决【2021】Multi-Source Anomaly Detection in Distributed IT Systems所遇到的程序开发问题。
如果觉得靠谱客网站的内容还不错,欢迎将靠谱客网站推荐给程序员好友。
发表评论 取消回复