概述
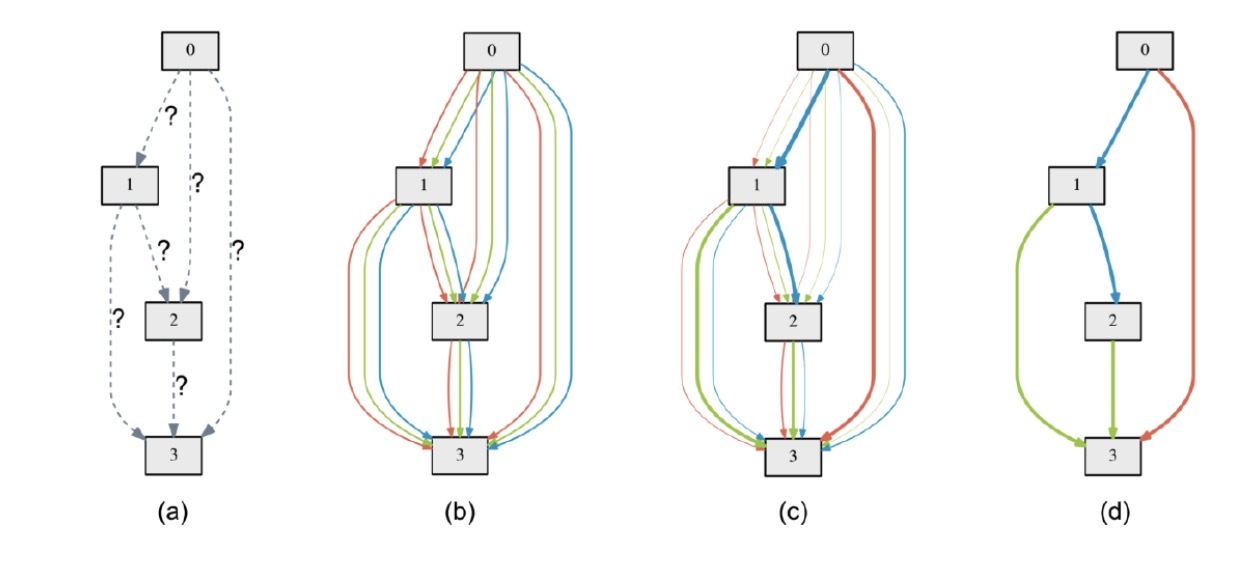
正如我之前的ResNet笔记一样,这篇文章主要是记录一个自己在阅读darts这篇论文时的过程,所得,以及踩过的一些坑。如果能帮助到大家,那就更好了。我学机器学习是在国外读的硕士,本身现在也在外企工作,有时候用词已经习惯了中英混杂,反而有时候强迫自己用全中文可能表达不太准确,请别介意。
该paper的标题为:DARTS: Differentiable Architecture Search, 也就是可微架构搜索,如果大家看不懂英文版,我在网上看到几篇还过得去的翻译,可以对照着看看。as follows:
- 一个比较简单的总结
- 来自知乎的翻译
这两个链接主要是翻译文章,如果英文过得去的同学完全没必要看,因为毕竟是翻译,一些翻译用词可能不符合自己的习惯,反而可能看不懂,还不如去看原文。好了,接下来笔记开始
一、神经网络架构搜索相关概念
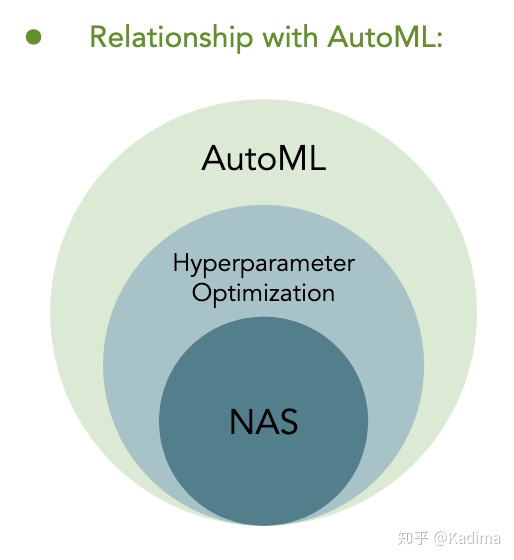
神经网络架构搜索,英文全称neural architecture search, 是AutoML的子类,主要focus on对于神经网络架构及其超参数的搜索。而AutoML包含的范围更广阔,比如有些人认为AutoML还应该包含自动数据清洗等功能、模块。而NAS的任务单一,仅仅是对于一个深度神经网络的架构和超参数进行自动搜索,一定程度上避免了人工调参的繁复工作。
可看一下wiki上对NAS的一个简要的介绍:
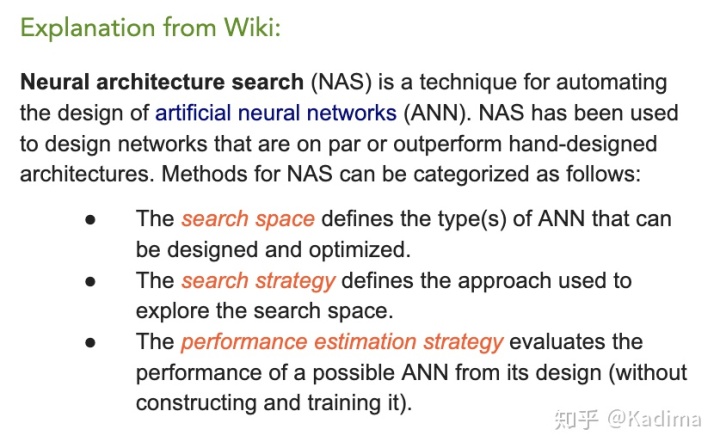
搜索空间可以理解为我要搭建一个神经网络,可用的积木、零件有哪些。而搜索策略通常为强化学习,基于梯度信息的优化,和贝叶斯优化等等。其中强化学习和基于梯度信息是目前比较火的搜索策略,比如ENAS就是用强化学习策略的一种架构索索方法。
ENAS:Efficient Neural Architecture Search via Parameter Sharing
然后,我们再来看一下NAS的一般流程:
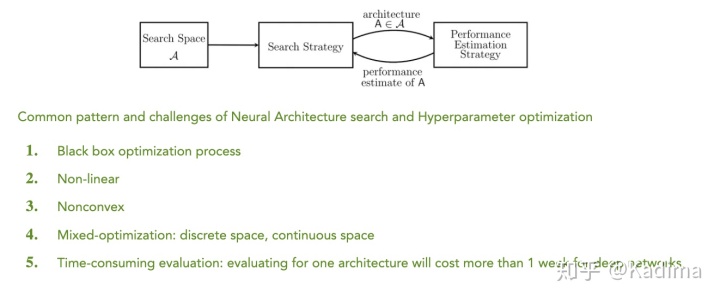
NAS的一般流程可以总结为上面这个简单的图。先定义一个搜索空间,然后通过一个搜索策略去不断地搭建模型-->预估表现-->根据预估表现再重新搭建模型-->...-->收敛。
有的人可能会问了,什么是预估表现?预估表现就是通过一定的方法去预先估计该架构到底行不行。再讲具体一点,我们训练一个深度神经网络,肯定要花费大量的时间去对一个网络训练到收敛(也就是达到某个局部最优解),而训练完之后,还要在验证集上验证模型的表现是否overfitting等,也是一个耗时的过程。由于完全地将一个网络训练好然后再去看它的表现这一步实在是太慢了(有的可能好几天,好几个星期)。而我们的目的是找一个好的架构,那避免不了要对n多个神经网络架构进行训练和看他们的表现。如果对于每一个根据搜索策略和搜索空间搭建出来的架构我们都走完这个完整的过程,那我们就别干活了。。。一年后见吧。
所以,我们就需要加速加速再加速这个过程,这就是预估表现被提出来放到这里的原因。对于加速预估模型表现的方法,一般有以下:
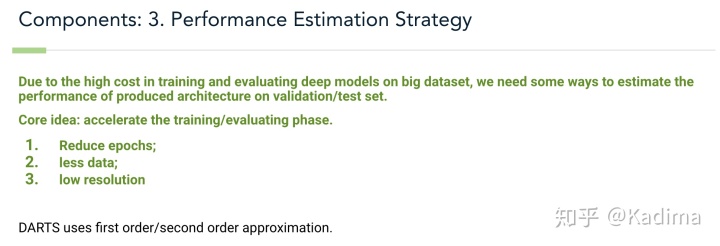
二、DARTS
介绍完了前期的基本概念后,可以开始正式的对该文章进行笔记了,首先放一个我对这篇文章总结的overview在这,让我们对文章有一个整体把握:Overview:
- Innovation
- Based on the continuous relaxation (by softmax) of the architecture representation, allowing efficient search of the architecture using gradient descent. (conventional approaches are applying evolution or reinforcement learning over a discrete and non-differentiable search space, which is a black box optimization)
- Dataset in experiment
- CV: Heavily train/evaluate on CIFAR-10. Transferable to ImageNet
- NLP: Heavily train/evaluate on Penn Treebank (PTB). Transferable to WikiText-2
- Performance
- Competitive network performance with much less params as well as search cost (GPU days) when compared to most RL/Evolution strategies.
DARTS和其他NAS算法在CIFAR-10上的结果
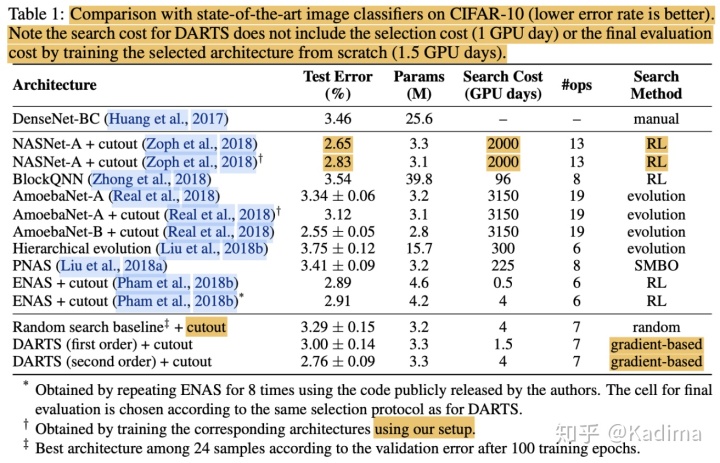
RL and Evolution are slow and they are black-box optimization.Inefficiency mainly caused by the black-box optimization problem over a discrete domain, which leads to a large number of architecture evaluations required. Such as: Based on RL, evolution, MCTS, SMBO, or Bayesian optimization.On the contrary, DARTS relaxes the discrete search space to be continuous, so that the architecture can be optimized with respect to its validation set performance by gradient descent. (using orders of magnitude fewer computation resources)
在这之前的现有的搜索算法,虽然能得到非常好的网络结构和超参数,但是计算消耗量太大,比如说,搜索到那时候在CIFAR-10和ImageNet上表现最好网络架构,如果用强化学习的搜索策略,需要2000 GPU days, 若用进化算法,则要用 3150 GPU days。尽管一些基于这两种策略的各种加速方法被提出,比如优化搜索结构,跨结构权值共享,但没有从根本上解决慢的问题。It also outperforms another recent efficient architecture search method ENAS.
特别地,DARTS 比很多现有的方法都要简单,它不含controllers,超网络,和性能预测器。
按文章原文来说:
Notably, DARTS is simpler than many existing approaches as it does not involve controllers (Zoph & Le, 2017; Baker et al., 2017; Zoph et al., 2018; Pham et al., 2018b; Zhong et al., 2018), hypernetworks (Brock et al., 2018) or performance predictors (Liu et al., 2018a), yet it is generic enough handle both convolutional and recurrent architectures.
其中的controllers实际上是一个rnn来基于现在的表现和结构,预测下一个模块的一个控制器(来自ENAS)。
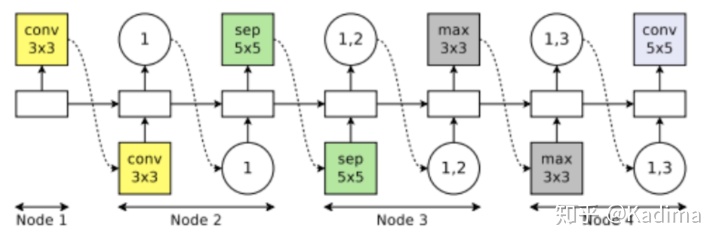
总的来说,这篇文章的contributions如下:
Contributions
- Bi-level optimization, applicable on both convolutional and recurrent architectures
- Achieve highly competitive results
- Achieve remarkable efficiency improvement
- Architecture learned by DARTS is transferable.
三、DARTS的搜索空间
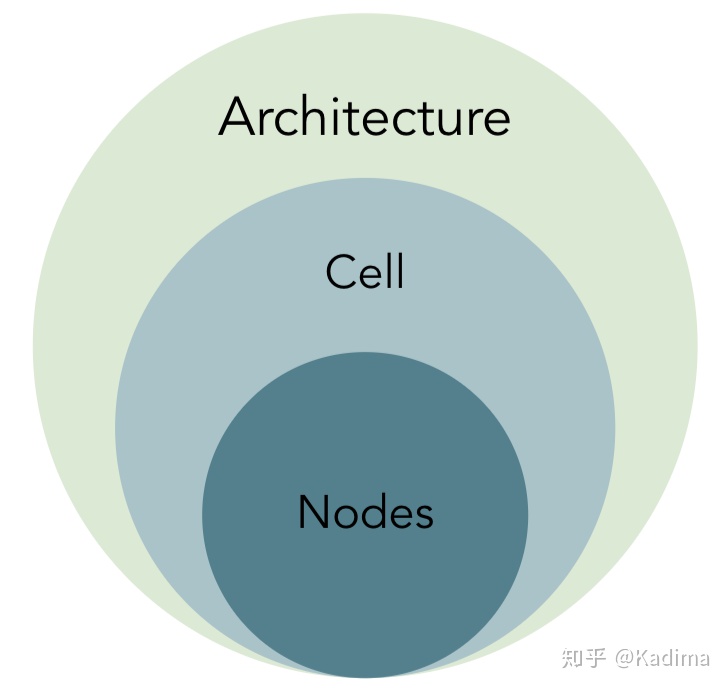
Darts的结构关系可以按上图所示进行理解。首先需要明确的是,Darts的结果本身是一个Architecture(架构),架构内部是一定的预先定义好数量的cell(单元),而每个cell内部是一些预先定义好数量的Nodes,而我们要学的东西,是Nodes及其连接方式。而每一个Nodes就可认为是一个操作或者积木。
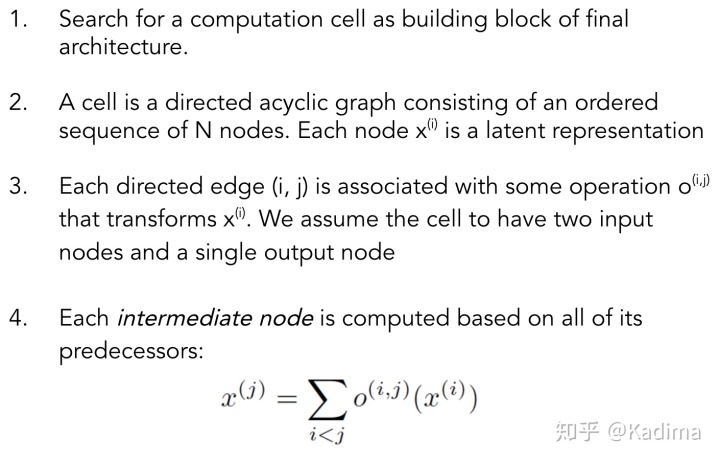
对于RNN和CNN的cell,每个cell内的第一个node叫input nodes。对于CNN来说,input为前两层的输出,而对于RNN来说,对应的是新的input和之前的”状态“(state)
For convolutional cells, the input nodes are defined as the cell outputs in the previous two layers. For recurrent cells, these are defined as the input at the current step and the state carried from the previous step. The output of the cell is obtained by applying a reduction operation (e.g. concatenation) to all the intermediate nodes.
在计算每一个node的操作时,它的操作被它之前所有的nodes(同一cell内)共同决定,如上述第4步所示的公式。
接下来,我们看看cell内部的nodes到底什么样:
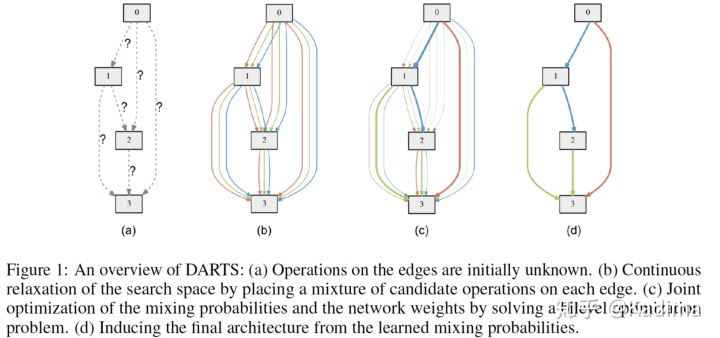
这是一个cell内部怎么运作的示意图。每个方块是一个node,对于一个node,它最开始是和之前所有node都连接起来,每一条线代表一个操作,比如在图像领域,可能就是 max pooling, convolution filter等。像soft EM一样,最开始保留所有的操作(也就是一个联合概率分布),然后不断地去根据training data 和 validation data 更新架构参数,直到收敛,然后保留概率值最大的操作。(也就是,最终,node和node之间,最多有一条线进行连接)
Notes:
- Every 2 nodes will only keep a single operation finally.
- A node will only connect to the previous top-k operations of distinct nodes. The strength is defined as follows (In the experiments, k = 2 in conv and k =1 in RNN)
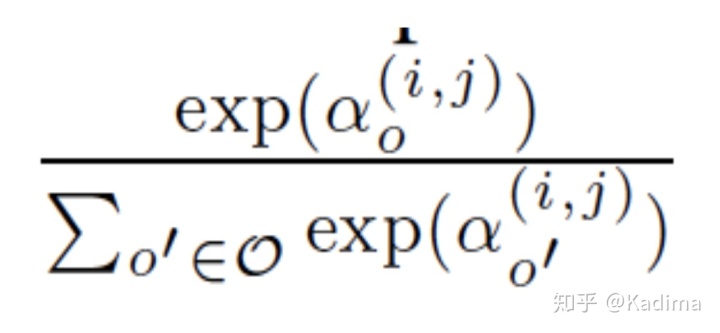
- A special zero operation is also included to indicate a lack of connection between two nodes. The task of learning the cell, therefore, reduces to learning the operations on its edges.
整篇文章的核心也就是将离散的搜索空间连续化,挺好懂的直接放个截图:
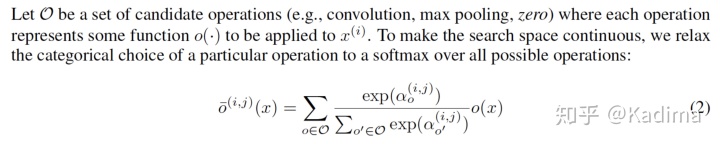
那么目标函数和优化方法是什么呢?
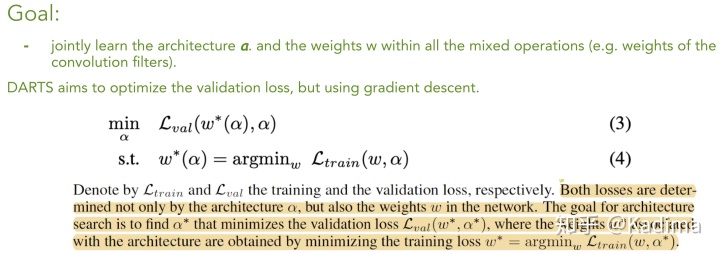
这里可以解释一下,a是一个架构参数,w*(a)是在某个具体的架构参数设定下的最优网络权重。所以目标函数为公式(3),同时需满足公式(4)。文章中也说了,(3)和(4)都依赖于w和a。
具体步骤:
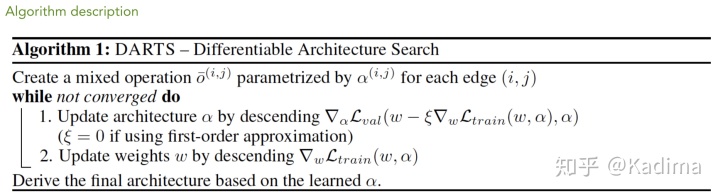
whereapproximate w*( α) by adapting w using only a single training step, without solving the inner optimization (equation 4) completely by training until convergence.denotes the current weights maintained by the algorithm, and ξ is the learning rate for a step of inner optimization. The idea is to
Similar ideas are also applied by:
Chelsea Finn, Pieter Abbeel, and Sergey Levine. Model-agnostic meta-learning for fast adaptation of deep networks. In ICML, pp. 1126–1135, 2017.
Jelena Luketina, Mathias Berglund, Klaus Greff, and Tapani Raiko. Scalable gradient-based tuning of continuous regularization hyperparameters. In ICML, pp. 2952–2960, 2016.
Luke Metz, Ben Poole, David Pfau, and Jascha Sohl-Dickstein. Unrolled generative adversarial networks. ICLR, 2017.
在这里我稍微做一下翻译和解释:
1. 对node中的每一条边,建立一系列混合的操作
持续执行以下两个步骤直到收敛:
1. 用所示公式更新架构参数
2. 根据该
根据所学的
根据上面的步骤描述,我们可以看见其实更新过程就是不断地去迭代优化
对于每个specific
那
https://github.com/quark0/darts/issues/101
这里,有两个需要回答的重要问题:
- DARTS是否保证收敛?
- DARTS找到的是否为全局最优解?
对于第一个问题,引用原文的回答:
While we are not currently aware of the convergence guarantees for our optimization algorithm, in practice it is able to reach a fixed point with a suitable choice of ksai(ξ)
对于第二个问题,答案是No
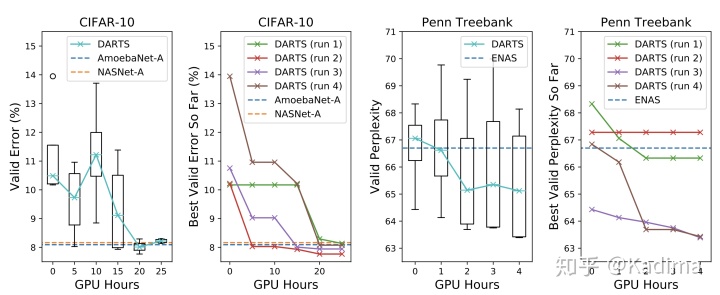
Figure 3: Search progress of DARTS for convolutional cells on CIFAR-10 and recurrent cells on Penn Treebank. We keep track of the most recent architectures over time. Each architecture snapshot is re-trained from scratch using the training set (for 100 epochs on CIFAR-10 and for 300 epochs on PTB) and then evaluated on the validation set. For each task, we repeat the experiments for 4 times with different random seeds, and report the median and the best (per run) validation performance of the architectures over time. As references, we also report the results (under the same evaluation setup; with comparable number of parameters) of the best existing cells discovered using RL or evolution, including NASNet-A (Zoph et al., 2018) (2000 GPU days), AmoebaNet-A (3150 GPU days) (Real et al., 2018) and ENAS (0.5 GPU day) (Pham et al., 2018b).
As references, we also report the results (under the same evaluation setup; with comparable number of parameters) of the best existing cells discovered using RL or evolution, including NASNet-A (Zoph et al., 2018) (2000 GPU days), AmoebaNet-A (3150 GPU days) (Real et al., 2018) and ENAS (0.5 GPU day) (Pham et al., 2018b).
----未完待续
2020.05.22 闲着无聊又来更新一下
由Figure 3, 我们可见 在图像问题上DARTS还是不那么依赖于初始化,(四次run都到了差不多的结果),而对于nlp问题,就非常依赖于初始化了。可见四条线的最终位置差距还是很大的(在y轴上)
推导细节:
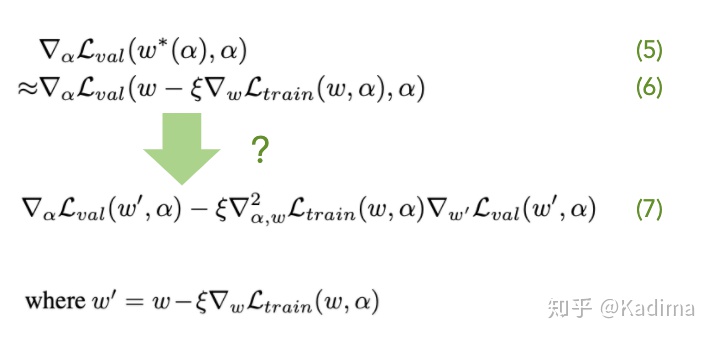
我在这个推导上卡了很久。。。为什么(6)式和(7)式的第一项长得一模一样?在此停留一段空白给大家思考一下,如果不明白的一定去看看维基百科对于链式法则的讲解!!(我一开始以为我懂什么是链式法则。。后来看完发现我是too young too simple)https://en.wikipedia.org/wiki/Chain_rule
。。。
在此我推荐一位大佬的解答:https://zhuanlan.zhihu.com/p/73037439
First-Order Approximation v.s. Second-Order Approximation

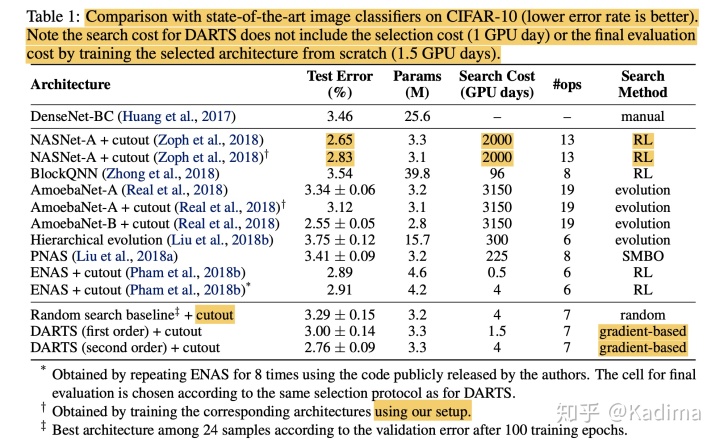
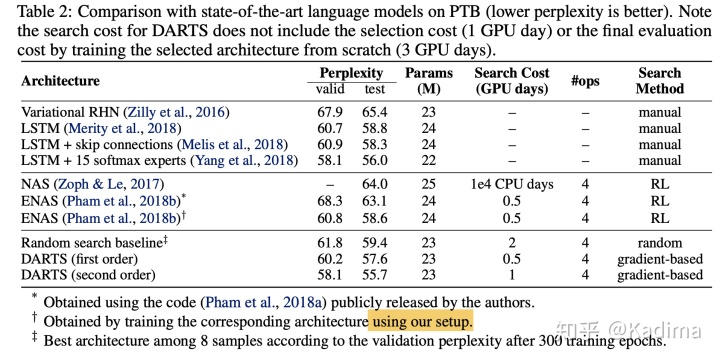
可见,second order approximation 结果要比 first order 好一些。
此外,作者们还做了一个小实验,如下图,设计了两个loss function
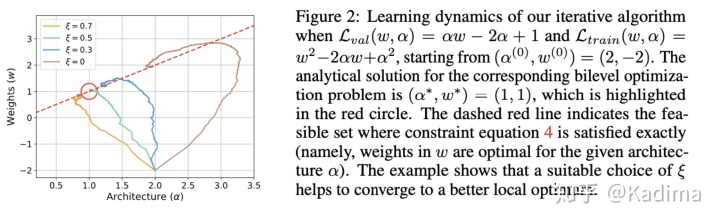
可见,合适的
到此结束啦,thanks for reading.
最后
以上就是碧蓝云朵为你收集整理的wsdl 架构验证警告:来自命名空间_Let it go: DARTS 神经网络可微架构搜索 笔记的全部内容,希望文章能够帮你解决wsdl 架构验证警告:来自命名空间_Let it go: DARTS 神经网络可微架构搜索 笔记所遇到的程序开发问题。
如果觉得靠谱客网站的内容还不错,欢迎将靠谱客网站推荐给程序员好友。
发表评论 取消回复