概述
文章目录
- 论文信息
- Xception 设计进化
- 深度可分离卷积 Depthwise Separable Convolution
- Xception 网络架构
- Xception 微调
论文信息
原文地址: Xception:Deep Learning with Depthwise Separable Convolutions
作者:François Chollet
Xception 设计进化
- Xception 是 Google 继 Inception 后提出的对 Inception-v3 的另一种改进。
- 作者认为,通道之间的相关性 与 空间相关性 最好要分开处理。采用 Separable Convolution(极致的 Inception 模块)来替换原来 Inception-v3中的卷积操作。
结构的变形过程如下:
- 在 Inception 中,特征可以通过 1 × 1 1×1 1×1 卷积, 3 × 3 3×3 3×3 卷积, 5 × 5 5×5 5×5 卷积,pooling 等进行提取,Inception 结构将特征类型的选择留给网络自己训练,也就是将一个输入同时输给几种提取特征方式,然后做 concat 。Inception-v3的结构图如下:
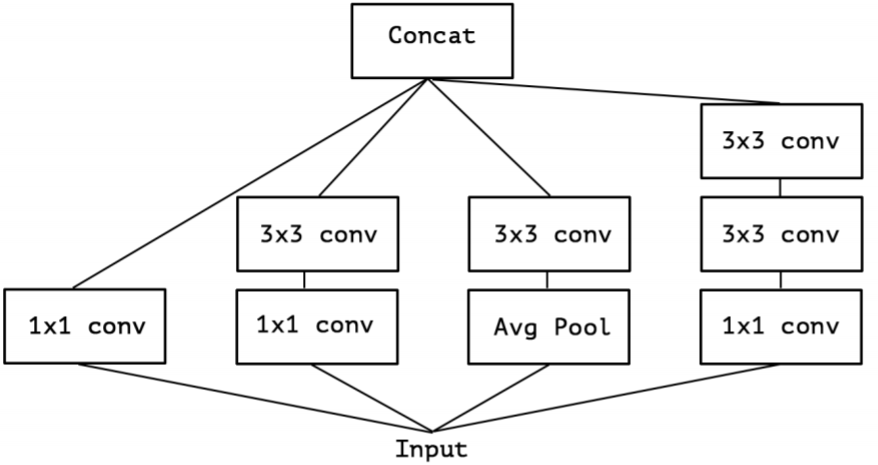
- 对 Inception-v3 进行简化,去除 Inception-v3 中的 avg pool 后,输入的下一步操作就都是 1 × 1 1×1 1×1 卷积:
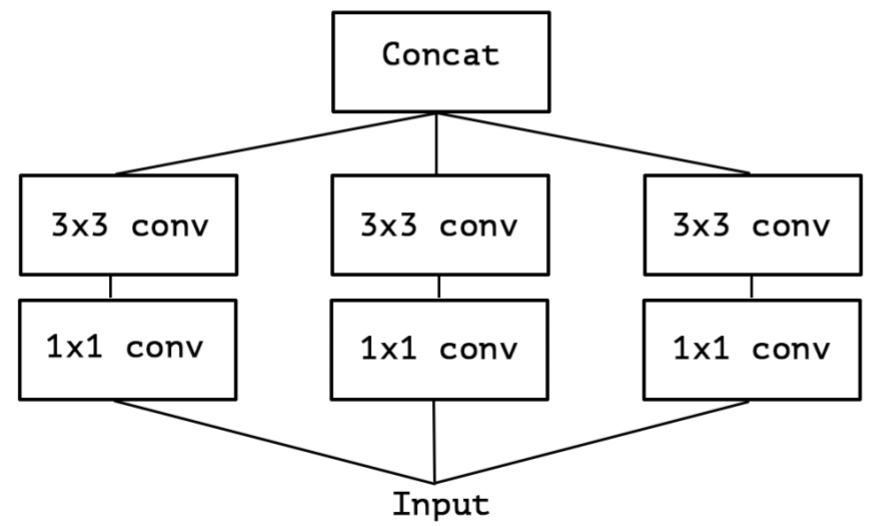
- 提取 1 × 1 1×1 1×1 卷积的公共部分:
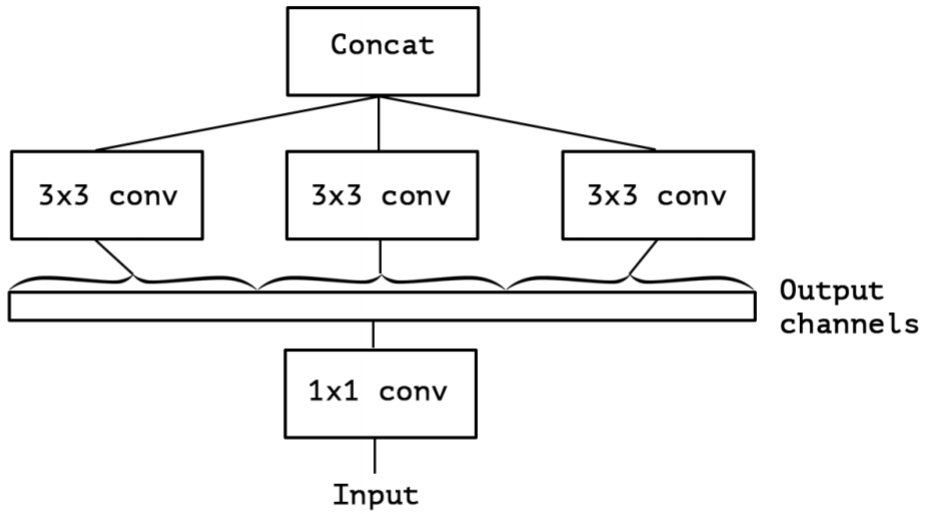
- Xception(极致的 Inception):先进行普通卷积操作,再对 1 × 1 1×1 1×1 卷积后的每个channel分别进行 3 × 3 3×3 3×3 卷积操作,最后将结果 concat:
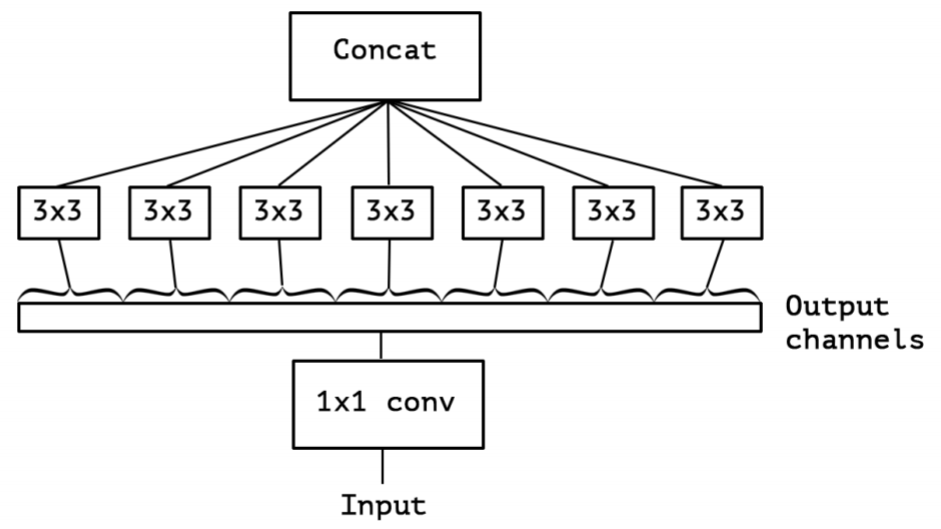
深度可分离卷积 Depthwise Separable Convolution
传统卷积的实现过程:
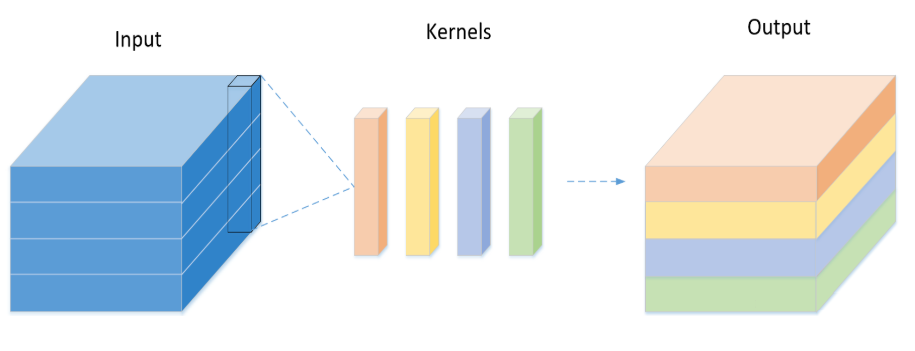
Depthwise Separable Convolution 的实现过程:
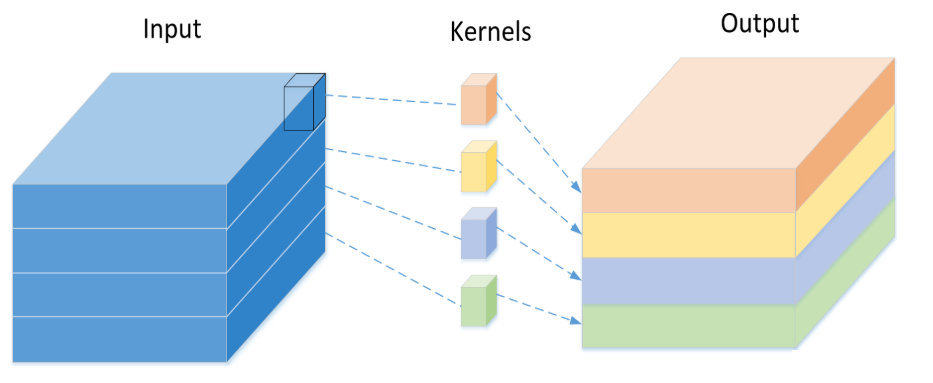
Depthwise Separable Convolution 与 极致的 Inception 区别:
-
极致的 Inception:
-
第一步:普通 1 × 1 1×1 1×1 卷积。
-
第二步:对 1 × 1 1×1 1×1 卷积结果的每个 channel,分别进行 3 × 3 3×3 3×3 卷积操作,并将结果 concat。
-
-
Depthwise Separable Convolution:
-
第一步:Depthwise 卷积,对输入的每个channel,分别进行 3 × 3 3×3 3×3 卷积操作,并将结果 concat。
-
第二步:Pointwise 卷积,对 Depthwise 卷积中的 concat 结果,进行 1 × 1 1×1 1×1 卷积操作。
-
两种操作的循序不一致:Inception 先进行 1 × 1 1×1 1×1 卷积,再进行 3 × 3 3×3 3×3 卷积;Depthwise Separable Convolution 先进行 3 × 3 3×3 3×3 卷积,再进行 1 × 1 1×1 1×1 卷积。(作者认为这个差异并没有大的影响)
作者发现,在“极致的 Inception”模块中,用于学习空间相关性的 3 × 3 3×3 3×3 的卷积,和用于学习通道间相关性的 1 × 1 1×1 1×1 卷积之间,不使用非线性激活函数时,收敛过程更快、准确率更高:
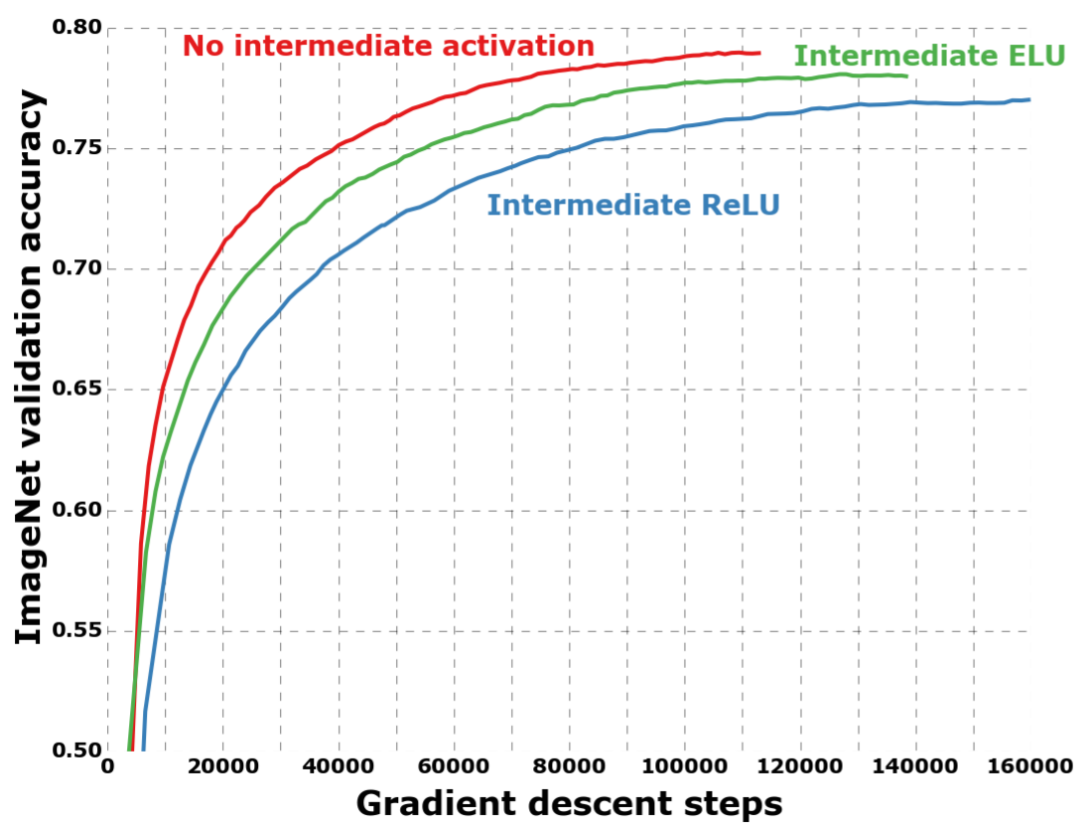
Xception 网络架构
Xception 的结构基于 ResNet,但是将其中的卷积层换成了Separable Convolution(极致的 Inception模块)。如下图所示。整个网络被分为了三个部分:Entry,Middle和Exit。
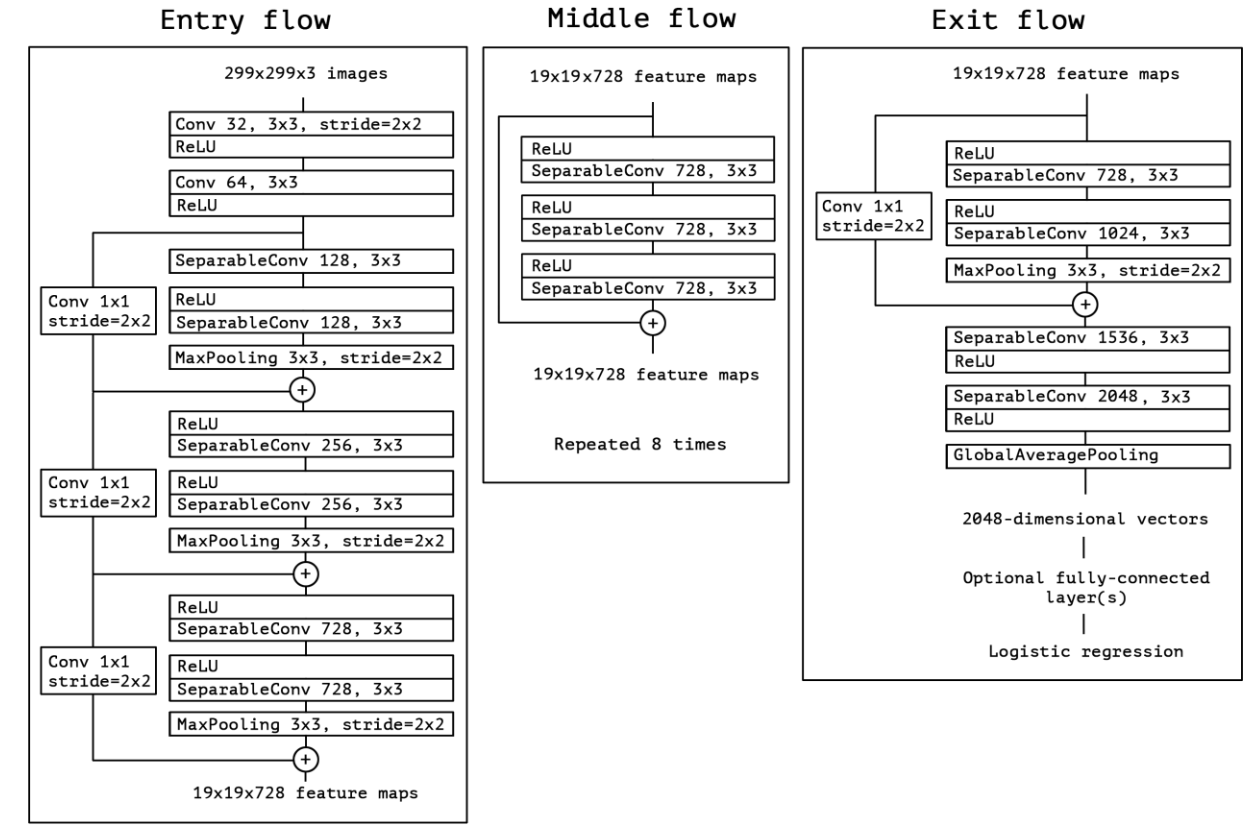
-
Xception 在 ImageNet 上,比 Inception-v3 的准确率稍高, 同时参数量有所下降,在 Xception 中加入的类似 ResNet 的残差连接机制也显著加快了Xception的收敛过程并获得了显著更高的准确率。
-
潜在的问题是,虽然 Depthwise Separable Convolution 可以带来准确率的提升或是理论计算量的大幅下降,但由于其计算过程较为零散,现有的卷积神经网络实现中它的效率都不够高,例如本文中 Xception 的理论计算量是远小于Inception-v3的,但其训练时的迭代速度反而更慢一些。
Xception 微调
Xception 权重文件下载:地址
from keras.models import Model
from keras import layers
from keras.layers import Dense, Input, BatchNormalization, Activation
from keras.layers import Conv2D, SeparableConv2D, MaxPooling2D, GlobalAveragePooling2D
from keras.optimizers import SGD
from sklearn.metrics import log_loss
from get_traffic_dataset import TrafficImageDataGenerator
train_file = './citySpace/outData/train/'
val_file = './citySpace/outData/val/'
def Xception(img_rows, img_cols, color_type,num_classes,weights_path = 'xception_weights_tf_dim_ordering_tf_kernels.h5'):
img_input = Input(shape=(img_rows, img_cols, color_type))
# Block 1
x = Conv2D(32, (3, 3), strides=(2, 2), use_bias=False)(img_input)
x = BatchNormalization()(x)
x = Activation('relu')(x)
x = Conv2D(64, (3, 3), use_bias=False)(x)
x = BatchNormalization()(x)
x = Activation('relu')(x)
residual = Conv2D(128, (1, 1), strides=(2, 2), padding='same', use_bias=False)(x)
residual = BatchNormalization()(residual)
# Block 2
x = SeparableConv2D(128, (3, 3), padding='same', use_bias=False)(x)
x = BatchNormalization()(x)
x = Activation('relu')(x)
x = SeparableConv2D(128, (3, 3), padding='same', use_bias=False)(x)
x = BatchNormalization()(x)
# Block 2 Pool
x = MaxPooling2D((3, 3), strides=(2, 2), padding='same')(x)
x = layers.add([x, residual])
residual = Conv2D(256, (1, 1), strides=(2, 2), padding='same', use_bias=False)(x)
residual = BatchNormalization()(residual)
# Block 3
x = Activation('relu')(x)
x = SeparableConv2D(256, (3, 3), padding='same', use_bias=False)(x)
x = BatchNormalization()(x)
x = Activation('relu')(x)
x = SeparableConv2D(256, (3, 3), padding='same', use_bias=False)(x)
x = BatchNormalization()(x)
# Block 3 Pool
x = MaxPooling2D((3, 3), strides=(2, 2), padding='same')(x)
x = layers.add([x, residual])
residual = Conv2D(728, (1, 1), strides=(2, 2), padding='same', use_bias=False)(x)
residual = BatchNormalization()(residual)
# Block 4
x = Activation('relu')(x)
x = SeparableConv2D(728, (3, 3), padding='same', use_bias=False)(x)
x = BatchNormalization()(x)
x = Activation('relu')(x)
x = SeparableConv2D(728, (3, 3), padding='same', use_bias=False)(x)
x = BatchNormalization()(x)
x = MaxPooling2D((3, 3), strides=(2, 2), padding='same')(x)
x = layers.add([x, residual])
# Block 5 - 12
for i in range(8):
residual = x
x = Activation('relu')(x)
x = SeparableConv2D(728, (3, 3), padding='same', use_bias=False)(x)
x = BatchNormalization()(x)
x = Activation('relu')(x)
x = SeparableConv2D(728, (3, 3), padding='same', use_bias=False)(x)
x = BatchNormalization()(x)
x = Activation('relu')(x)
x = SeparableConv2D(728, (3, 3), padding='same', use_bias=False)(x)
x = BatchNormalization()(x)
x = layers.add([x, residual])
residual = Conv2D(1024, (1, 1), strides=(2, 2), padding='same', use_bias=False)(x)
residual = BatchNormalization()(residual)
# Block 13
x = Activation('relu')(x)
x = SeparableConv2D(728, (3, 3), padding='same', use_bias=False)(x)
x = BatchNormalization()(x)
x = Activation('relu')(x)
x = SeparableConv2D(1024, (3, 3), padding='same', use_bias=False)(x)
x = BatchNormalization()(x)
# Block 13 Pool
x = MaxPooling2D((3, 3), strides=(2, 2), padding='same')(x)
x = layers.add([x, residual])
# Block 14
x = SeparableConv2D(1536, (3, 3), padding='same', use_bias=False)(x)
x = BatchNormalization()(x)
x = Activation('relu')(x)
# Block 14 part 2
x = SeparableConv2D(2048, (3, 3), padding='same', use_bias=False)(x)
x = BatchNormalization()(x)
x = Activation('relu')(x)
# Fully Connected Layer
x_fc = GlobalAveragePooling2D()(x)
x_fc = Dense(1000, activation='softmax')(x_fc)
inputs = img_input
# Create model
model = Model(inputs, x_fc, name='xception')
# load weights
model.load_weights(weights_path)
# Truncate and replace softmax layer for transfer learning
# Cannot use model.layers.pop() since model is not of Sequential() type
# The method below works since pre-trained weights are stored in layers but not in the model
x_newfc = GlobalAveragePooling2D()(x)
x_newfc = Dense(num_classes, activation='softmax', name='fc_out')(x_newfc)
# Create another model with our customized softmax
model = Model(img_input, x_newfc)
# Learning rate is changed to 0.001
sgd = SGD(lr=1e-3, decay=1e-6, momentum=0.9, nesterov=True)
model.compile(optimizer=sgd, loss='categorical_crossentropy', metrics=['accuracy'])
return model
if __name__ == '__main__':
img_rows, img_cols = 299, 299 # Resolution of inputs
channel = 3
num_classes = 3
batch_size = 16
nb_epoch = 5
# Initalize the data generator seperately for the training and validation set
train_generator = TrafficImageDataGenerator(train_file, scale_size=(img_rows, img_cols), horizontal_flip=True, shuffle=True)
val_generator = TrafficImageDataGenerator(val_file, scale_size=(img_rows, img_cols), horizontal_flip=True, shuffle=True)
X_valid, Y_valid,val_labels = val_generator.all(1000)
X_train, Y_train, train_labels = train_generator.all(5000)
# Load our model
model = Xception(img_rows, img_cols,channel,num_classes)
# Start Fine-tuning
model.fit(X_train, Y_train,
batch_size=batch_size,
nb_epoch=nb_epoch,
shuffle=True,
verbose=1,
validation_data=(X_valid, Y_valid),
)
# Make predictions
predictions_valid = model.predict(X_valid, batch_size=batch_size, verbose=1)
# Cross-entropy loss score
score = log_loss(Y_valid, predictions_valid)
最后
以上就是舒服小天鹅为你收集整理的Xception的全部内容,希望文章能够帮你解决Xception所遇到的程序开发问题。
如果觉得靠谱客网站的内容还不错,欢迎将靠谱客网站推荐给程序员好友。
发表评论 取消回复