概述
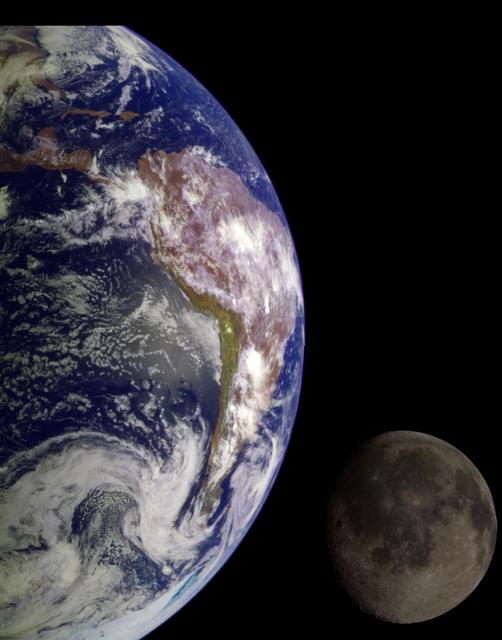
由于生产需要、项目组需要,需要将之前神经网络训练的模型(keras模型hdf5类型),转换成在window环境下C++能够调用的类型。
Tensorflow支持windows环境,而且可以被vs2010调用。
在配置好windows + tensorflow的前提下,需要将Keras(*.h)模型文件转换为Tensorflow(*.pb)模型文件,便于相关api调用模型属性。
主要参考一篇教程
amir-abdi/keras_to_tensorflowgithub.com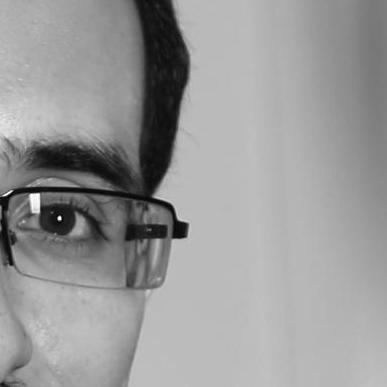
import tensorflow as tf
from tensorflow.python.framework import graph_util
from tensorflow.python.framework import graph_io
from pathlib import Path
from absl import app
from absl import flags
from absl import logging
import keras
from keras import backend as K
from keras.models import model_from_json, model_from_yaml
K.set_learning_phase(0)
FLAGS = flags.FLAGS
flags.DEFINE_string('input_model', None, 'Path to the input model.')
flags.DEFINE_string('input_model_json', None, 'Path to the input model '
'architecture in json format.')
flags.DEFINE_string('input_model_yaml', None, 'Path to the input model '
'architecture in yaml format.')
flags.DEFINE_string('output_model', None, 'Path where the converted model will '
'be stored.')
flags.DEFINE_boolean('save_graph_def', False,
'Whether to save the graphdef.pbtxt file which contains '
'the graph definition in ASCII format.')
flags.DEFINE_string('output_nodes_prefix', None,
'If set, the output nodes will be renamed to '
'`output_nodes_prefix`+i, where `i` will numerate the '
'number of of output nodes of the network.')
flags.DEFINE_boolean('quantize', False,
'If set, the resultant TensorFlow graph weights will be '
'converted from float into eight-bit equivalents. See '
'documentation here: '
'https://github.com/tensorflow/tensorflow/tree/master/tensorflow/tools/graph_transforms')
flags.DEFINE_boolean('channels_first', False,
'Whether channels are the first dimension of a tensor. '
'The default is TensorFlow behaviour where channels are '
'the last dimension.')
flags.DEFINE_boolean('output_meta_ckpt', False,
'If set to True, exports the model as .meta, .index, and '
'.data files, with a checkpoint file. These can be later '
'loaded in TensorFlow to continue training.')
flags.mark_flag_as_required('input_model')
flags.mark_flag_as_required('output_model')
def load_model(input_model_path, input_json_path=None, input_yaml_path=None):
if not Path(input_model_path).exists():
raise FileNotFoundError(
'Model file `{}` does not exist.'.format(input_model_path))
try:
model = keras.models.load_model(input_model_path)
return model
except FileNotFoundError as err:
logging.error('Input mode file (%s) does not exist.', FLAGS.input_model)
raise err
except ValueError as wrong_file_err:
if input_json_path:
if not Path(input_json_path).exists():
raise FileNotFoundError(
'Model description json file `{}` does not exist.'.format(
input_json_path))
try:
model = model_from_json(open(str(input_json_path)).read())
model.load_weights(input_model_path)
return model
except Exception as err:
logging.error("Couldn't load model from json.")
raise err
elif input_yaml_path:
if not Path(input_yaml_path).exists():
raise FileNotFoundError(
'Model description yaml file `{}` does not exist.'.format(
input_yaml_path))
try:
model = model_from_yaml(open(str(input_yaml_path)).read())
model.load_weights(input_model_path)
return model
except Exception as err:
logging.error("Couldn't load model from yaml.")
raise err
else:
logging.error(
'Input file specified only holds the weights, and not '
'the model definition. Save the model using '
'model.save(filename.h5) which will contain the network '
'architecture as well as its weights. '
'If the model is saved using the '
'model.save_weights(filename) function, either '
'input_model_json or input_model_yaml flags should be set to '
'to import the network architecture prior to loading the '
'weights. n'
'Check the keras documentation for more details '
'(https://keras.io/getting-started/faq/)')
raise wrong_file_err
def main(args):
# If output_model path is relative and in cwd, make it absolute from root
output_model = FLAGS.output_model
if str(Path(output_model).parent) == '.':
output_model = str((Path.cwd() / output_model))
output_fld = Path(output_model).parent
output_model_name = Path(output_model).name
output_model_stem = Path(output_model).stem
output_model_pbtxt_name = output_model_stem + '.pbtxt'
# Create output directory if it does not exist
Path(output_model).parent.mkdir(parents=True, exist_ok=True)
if FLAGS.channels_first:
K.set_image_data_format('channels_first')
else:
K.set_image_data_format('channels_last')
model = load_model(FLAGS.input_model, FLAGS.input_model_json, FLAGS.input_model_yaml)
# TODO(amirabdi): Support networks with multiple inputs
orig_output_node_names = [node.op.name for node in model.outputs]
if FLAGS.output_nodes_prefix:
num_output = len(orig_output_node_names)
pred = [None] * num_output
converted_output_node_names = [None] * num_output
# Create dummy tf nodes to rename output
for i in range(num_output):
converted_output_node_names[i] = '{}{}'.format(
FLAGS.output_nodes_prefix, i)
pred[i] = tf.identity(model.outputs[i],
name=converted_output_node_names[i])
else:
converted_output_node_names = orig_output_node_names
logging.info('Converted output node names are: %s',
str(converted_output_node_names))
sess = K.get_session()
if FLAGS.output_meta_ckpt:
saver = tf.train.Saver()
saver.save(sess, str(output_fld / output_model_stem))
if FLAGS.save_graph_def:
tf.train.write_graph(sess.graph.as_graph_def(), str(output_fld),
output_model_pbtxt_name, as_text=True)
logging.info('Saved the graph definition in ascii format at %s',
str(Path(output_fld) / output_model_pbtxt_name))
if FLAGS.quantize:
from tensorflow.tools.graph_transforms import TransformGraph
transforms = ["quantize_weights", "quantize_nodes"]
transformed_graph_def = TransformGraph(sess.graph.as_graph_def(), [],
converted_output_node_names,
transforms)
constant_graph = graph_util.convert_variables_to_constants(
sess,
transformed_graph_def,
converted_output_node_names)
else:
constant_graph = graph_util.convert_variables_to_constants(
sess,
sess.graph.as_graph_def(),
converted_output_node_names)
graph_io.write_graph(constant_graph, str(output_fld), output_model_name,
as_text=False)
logging.info('Saved the freezed graph at %s',
str(Path(output_fld) / output_model_name))
if __name__ == "__main__":
app.run(main)
运行如下代码:
python keras_to_tensorflow.py --input_model="path/to/keras/model.h5" --input_model_json="path/to/keras/model.json" --output_model="path/to/save/model.pb"
最后
以上就是积极雨为你收集整理的转换pb_Keras模型转换成Tensorflow模型方法的全部内容,希望文章能够帮你解决转换pb_Keras模型转换成Tensorflow模型方法所遇到的程序开发问题。
如果觉得靠谱客网站的内容还不错,欢迎将靠谱客网站推荐给程序员好友。
本图文内容来源于网友提供,作为学习参考使用,或来自网络收集整理,版权属于原作者所有。
发表评论 取消回复