概述
???? Author :Horizon Max
✨ 编程技巧篇:各种操作小结
???? 机器视觉篇:会变魔术 OpenCV
???? 深度学习篇:简单入门 PyTorch
???? 神经网络篇:经典网络模型
???? 算法篇:再忙也别忘了 LeetCode
[ 图像分类 ] 经典网络模型实例—— CIFAR10 + ResNet50 详解与复现
- ???? CIFAR10 + ResNet50 图像分类实例
- ???? 准备工作
- ???? 库文件准备
- ???? 命令行接口
- ???? 加载数据集
- ???? 加载模型
- ???? 定义损失函数与优化器
- ???? 打印模型
- ???? 模型训练与测试
- ???? 模型训练
- ???? 模型测试
- ???? 训练过程数据分析
- ???? 保存模型
- ???? 保存训练参数
- ???? 绘制 Loss-Acc 曲线
- ???? 主函数
- ???? CIFAR10 + ResNet50 完整代码
???? CIFAR10 + ResNet50 图像分类实例
本篇博客具体介绍如何使用 ResNet50
网络实现 CIFAR-10
数据集的分类 ;
相关 python库 版本:
python 3.6
pytorch 1.8.0
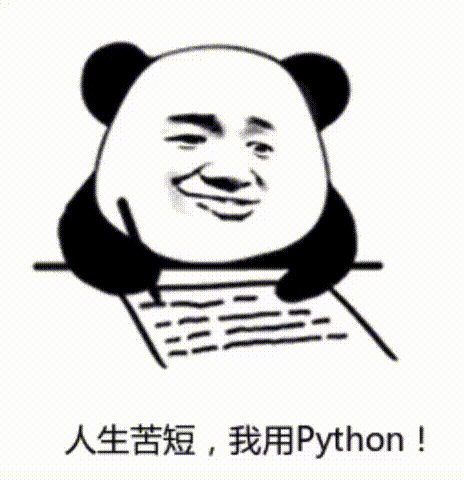
CIFAR-10 博客介绍 ???? :[ 数据集 ] CIFAR-10数据集介绍
ResNet50 博客介绍 ???? :[ 图像分类 ] 经典网络模型4——ResNet 详解与复现
???? 准备工作
???? 库文件准备
from models import *
from torchinfo import summary
import torch.optim as optim
import torchvision
import torchvision.transforms as transforms
from torch.utils.data import DataLoader
import matplotlib.pyplot as plt
import os
import time
import pandas as pd
from datetime import datetime
from tqdm import tqdm
import argparse
创建 models
文件夹用于存放各种 网络模型 :
from models import *
其中 __init__.py
:
from .AlexNet import *
from .VGG import *
from .GoogLeNet import *
from .ResNet import *
from .DenseNet import *
from .SENet import *
from .CBAM import *
from .ECANet import *
from .SqueezeNet import *
from .MobileNet import *
from .ShuffleNet import *
from .Xception import *
所有的 网络模型 参考 ????:经典网络模型 —— 盘点 22篇必读论文与网络模型 + 5种常见数据集
记得将函数里面的 num_classes
改成 10
;
???? 命令行接口
使用 argparse.ArgumentParser()
函数 :
- 创建解析器
- 添加参数
- 解析参数
parser = argparse.ArgumentParser(description='Train CIFAR10 with PyTorch')
parser.add_argument('--lr', default=0.1, type=float, help='learning rate')
parser.add_argument('--resume', '-r', action='store_true',
help='resume from checkpoint')
args = parser.parse_args()
classes = ('plane', 'car', 'bird', 'cat', 'deer',
'dog', 'frog', 'horse', 'ship', 'truck')
Terminal
窗口输入 :
模型训练: python models_classification_pytorch.py
模型加载训练: python models_classification_pytorch.py --resume --lr=0.01
???? 加载数据集
这里使用的是 DataLoader
函数 :
batch_size = 128
transform_train = transforms.Compose([
transforms.RandomCrop(32, padding=2),
transforms.RandomHorizontalFlip(),
transforms.ToTensor(),
transforms.Normalize((0.4914, 0.4822, 0.4465), (0.2023, 0.1994, 0.2010)),
])
transform_test = transforms.Compose([
transforms.ToTensor(),
transforms.Normalize((0.4914, 0.4822, 0.4465), (0.2023, 0.1994, 0.2010)),
])
train_set = torchvision.datasets.CIFAR10(
root='./CIFAR10', train=True, download=False, transform=transform_train)
train_loader = torch.utils.data.DataLoader(
dataset=train_set, batch_size=batch_size, shuffle=True, num_workers=0)
test_set = torchvision.datasets.CIFAR10(
root='./CIFAR10', train=False, download=False, transform=transform_test)
test_loader = torch.utils.data.DataLoader(
dataset=test_set, batch_size=batch_size, shuffle=True, num_workers=0)
- 如果出现显存占满的问题,将
batch_size
改成64
即可 - 训练集处理时采用了
数据增强
的方法,包括:填充裁剪和水平翻转 Normalize
对输入的数据集进行归一化处理,后面的参数是在 ImageNet 数据集中实验得到的最佳参数- 如果没有下载数据集,修改参数:
download=Ture
???? 加载模型
net
为可供加载作为特征提取的神经网络模型 :
print('------ Check CNN Model ------')
# net = AlexNet()
# net = VGG16() # VGG11/13/16/19
# net = GoogLeNet()
# net = ResNet50() # ResNet18/34/50/101/152
# net = DenseNet121() # DenseNet121/161/169/201/264
# net = SE_ResNet50()
# net = CBAM_ResNet50()
# net = ECA_ResNet50()
# net = squeezenet1_0() # squeezenet1_0/1_1
# net = MobileNet()
# net = shufflenet_g8() # shufflenet_g1/g2/g3/g4/g8
# net = Xception()
best_acc = 0
start_epoch = 0
end_epoch = start_epoch + 200
net = ResNet50()
device = 'cuda' if torch.cuda.is_available() else 'cpu'
print('cuda is available : ', torch.cuda.is_available())
net = net.to(device)
if args.resume:
print('------ Loading checkpoint ------')
assert os.path.isdir('checkpoint'), 'Error: no checkpoint directory found!'
checkpoint = torch.load('./checkpoint/ckpt.pth')
net.load_state_dict(checkpoint['net'])
best_acc = checkpoint['acc']
start_epoch = checkpoint['epoch']
end_epoch += start_epoch
- 训练次数修改参数:
end_epoch
- 加载模型步骤:选择模型 —— 将模型传入
device
- 如果是重新加载训练,就会进去到
args.resume
中 - 修改加载的模型的地址:
'./checkpoint/ckpt.pth'
???? 定义损失函数与优化器
使用的是交叉熵损失函数 CrossEntropyLoss
与 SGD
优化器 :
criterion = nn.CrossEntropyLoss()
optimizer = optim.SGD(net.parameters(), lr=args.lr,
momentum=0.9, weight_decay=5e-4)
scheduler = torch.optim.lr_scheduler.StepLR(optimizer, 20, gamma=0.5)
- 初始学习率 0.1,学习动量 0.9,权重衰减 5e-4
- 每20个epoch,学习率变为原来的0.5倍,最终的学习率为 lr = 0.1 * 0.5(n-1)
???? 打印模型
使用定义的 model_summary
函数将模型结构与参数打印出来,方便查看 :
def model_summary():
print('------ Model Summary ------')
y = net(torch.randn(1, 3, 32, 32).to(device))
print(y.size())
summary(net, (1, 3, 32, 32), depth=5)
模型打印效果图 :
???? 模型训练与测试
???? 模型训练
注意设置参数 :net.train()
def train(epoch):
print('nEpoch: %d' % epoch)
net.train()
train_loss = 0
correct = 0
total = 0
for batch_idx, (inputs, targets) in enumerate(tqdm(train_loader)):
inputs, targets = inputs.to(device), targets.to(device)
optimizer.zero_grad()
outputs = net(inputs)
loss = criterion(outputs, targets)
loss.backward()
optimizer.step()
train_loss += loss.item()
_, predicted = outputs.max(1)
total += targets.size(0)
correct += predicted.eq(targets).sum().item()
if batch_idx % 50 == 0:
print('tLoss: %.3f | Acc: %.3f%% (%d/%d)'
% (train_loss / (batch_idx + 1), 100. * correct / total, correct, total))
train_loss = train_loss / len(train_loader)
train_acc = 100. * correct / total
print('n', time.asctime(time.localtime(time.time())))
print(' Epoch: %d | Train_loss: %.3f | Train_acc: %.3f%% n' % (epoch, train_loss, train_acc))
return train_loss, train_acc
- 图片
inputs
和 标签targets
传入device
- 每一个epoch训练都需要
梯度清零
,采用的是随机批量梯度下降
- 图片
inputs
和 标签targets
传入 模型net
- 计算损失
loss
- 反向传播 计算梯度
- 更新梯度
- 打印训练过程:loss 和 acc
训练过程 :
???? 模型测试
注意设置参数 :net.eval()
def test(epoch):
global best_acc
net.eval()
test_loss = 0
correct = 0
total = 0
with torch.no_grad():
for batch_idx, (inputs, targets) in enumerate(tqdm(test_loader)):
inputs, targets = inputs.to(device), targets.to(device)
outputs = net(inputs)
loss = criterion(outputs, targets)
test_loss += loss.item()
_, predicted = outputs.max(1)
total += targets.size(0)
correct += predicted.eq(targets).sum().item()
if batch_idx % 20 == 0:
print('tLoss: %.3f | Acc: %.3f%% (%d/%d)'
% (train_loss / (batch_idx + 1), 100. * correct / total, correct, total))
test_loss = test_loss / len(test_loader)
test_acc = 100. * correct / total
print('n', time.asctime(time.localtime(time.time())))
print(' Epoch: %d | Test_loss: %.3f | Test_acc: %.3f%% n' % (epoch, test_loss, test_acc))
return test_loss, test_acc
- 图片
inputs
和 标签targets
传入device
- 每一个epoch训练都需要
梯度清零
,采用的是随机批量梯度下降
- 图片
inputs
和 标签targets
传入 模型net
- 计算损失
loss
- 打印训练过程:loss 和 acc
测试过程 :
???? 训练过程数据分析
???? 保存模型
利用 best_acc
参数用于判断存放测试效果最好的模型 :
if test_acc > best_acc:
print('------ Saving model------')
state = {
'net': net.state_dict(),
'acc': test_acc,
'epoch': epoch,
}
if not os.path.isdir('checkpoint'):
os.mkdir('checkpoint')
torch.save(state, './checkpoint/model_%d_%.3f.pth' % (epoch, best_acc))
best_acc = test_acc
???? 保存训练参数
将训练过程的参数保存至 train_acc.csv
文件中 :
- epoch
- train_loss
- train_accuracy
- test_loss
- test_accuracy
def save_csv(epoch, save_train_loss, save_train_acc, save_test_loss, save_test_acc):
time = '%s' % datetime.now()
step = 'Step[%d]' % epoch
train_loss = '%f' % save_train_loss
train_acc = '%g' % save_train_acc
test_loss = '%f' % save_test_loss
test_acc = '%g' % save_test_acc
print('------ Saving csv ------')
list = [time, step, train_loss, train_acc, test_loss, test_acc]
data = pd.DataFrame([list])
data.to_csv('./train_acc.csv', mode='a', header=False, index=False)
保存的数据如下所示 :
???? 绘制 Loss-Acc 曲线
对保存的 train_acc.csv
文件进行数据读取与绘制 :
- epoch
- train_loss
- train_accuracy
- test_loss
- test_accuracy
def draw_acc():
filename = r'./train_acc.csv'
train_data = pd.read_csv(filename)
print(train_data.head())
length = len(train_data['step'])
Epoch = list(range(1, length + 1))
train_loss = train_data['train loss']
train_accuracy = train_data['train accuracy']
test_loss = train_data['test loss']
test_accuracy = train_data['test accuracy']
plt.plot(Epoch, train_loss, 'g-.', label='train loss')
plt.plot(Epoch, train_accuracy, 'r-', label='train accuracy')
plt.plot(Epoch, test_loss, 'b-.', label='test loss')
plt.plot(Epoch, test_accuracy, 'm-', label='test accuracy')
plt.xlabel('Epoch')
plt.ylabel('Loss & Accuracy')
plt.yticks([j for j in range(0, 101, 10)])
plt.title('Epoch -- Loss & Accuracy')
plt.legend(loc='center right', fontsize=8, frameon=False)
plt.show()
???? 主函数
- 打印网络模型
- 建立 csv 文件用于存放训练过程的参数
- 模型训练
- 模型测试
- 保存训练参数
- 绘制 Loss-Acc 曲线
if __name__ == '__main__':
model_summary()
df = pd.DataFrame(columns=['time', 'step', 'train loss', 'train accuracy', 'test loss', 'test accuracy'])
df.to_csv('./train_acc.csv', index=False)
for epoch in range(start_epoch, end_epoch):
train_loss, train_acc = train(epoch)
test_loss, test_acc = test(epoch)
scheduler.step()
save_csv(epoch, train_loss, train_acc, test_loss, test_acc)
draw_acc()
???? CIFAR10 + ResNet50 完整代码
以下是采用 ResNet50
对 CIFAR10
数据集进行分类的完整的代码 ;
可以修改网络模型实现其他网络的训练与分类 ;
# Here is the code :
######################################
# #
# Train CIFAR10 with PyTorch #
# #
######################################
from models import *
from torchinfo import summary
import torch.optim as optim
import torchvision
import torchvision.transforms as transforms
from torch.utils.data import DataLoader
import matplotlib.pyplot as plt
import os
import time
import pandas as pd
from datetime import datetime
from tqdm import tqdm
import argparse
parser = argparse.ArgumentParser(description='Train CIFAR10 with PyTorch')
parser.add_argument('--lr', default=0.1, type=float, help='learning rate')
parser.add_argument('--resume', '-r', action='store_true',
help='resume from checkpoint')
args = parser.parse_args()
classes = ('plane', 'car', 'bird', 'cat', 'deer',
'dog', 'frog', 'horse', 'ship', 'truck')
##########################
# Data #
##########################
print('------ Preparing data ------')
batch_size = 128
transform_train = transforms.Compose([
transforms.RandomCrop(32, padding=2),
transforms.RandomHorizontalFlip(),
transforms.ToTensor(),
transforms.Normalize((0.4914, 0.4822, 0.4465), (0.2023, 0.1994, 0.2010)),
])
transform_test = transforms.Compose([
transforms.ToTensor(),
transforms.Normalize((0.4914, 0.4822, 0.4465), (0.2023, 0.1994, 0.2010)),
])
train_set = torchvision.datasets.CIFAR10(
root='./CIFAR10', train=True, download=False, transform=transform_train)
train_loader = torch.utils.data.DataLoader(
dataset=train_set, batch_size=batch_size, shuffle=True, num_workers=0)
test_set = torchvision.datasets.CIFAR10(
root='./CIFAR10', train=False, download=False, transform=transform_test)
test_loader = torch.utils.data.DataLoader(
dataset=test_set, batch_size=batch_size, shuffle=True, num_workers=0)
##########################
# Model #
##########################
print('------ Check CNN Model ------')
# net = AlexNet()
# net = VGG16() # VGG11/13/16/19
# net = GoogLeNet()
# net = ResNet50() # ResNet18/34/50/101/152
# net = DenseNet121() # DenseNet121/161/169/201/264
# net = SE_ResNet50()
# net = CBAM_ResNet50()
# net = ECA_ResNet50()
# net = squeezenet1_0() # squeezenet1_0/1_1
# net = MobileNet()
# net = shufflenet_g8() # shufflenet_g1/g2/g3/g4/g8
# net = Xception()
best_acc = 0
start_epoch = 0
end_epoch = start_epoch + 200
net = ResNet50()
device = 'cuda' if torch.cuda.is_available() else 'cpu'
print('cuda is available : ', torch.cuda.is_available())
net = net.to(device)
if args.resume:
print('------ Loading checkpoint ------')
assert os.path.isdir('checkpoint'), 'Error: no checkpoint directory found!'
checkpoint = torch.load('./checkpoint/ckpt.pth')
net.load_state_dict(checkpoint['net'])
best_acc = checkpoint['acc']
start_epoch = checkpoint['epoch']
end_epoch += start_epoch
criterion = nn.CrossEntropyLoss()
optimizer = optim.SGD(net.parameters(), lr=args.lr,
momentum=0.9, weight_decay=5e-4)
scheduler = torch.optim.lr_scheduler.StepLR(optimizer, 20, gamma=0.5)
##########################
# Model Summary #
##########################
def model_summary():
print('------ Model Summary ------')
y = net(torch.randn(1, 3, 32, 32).to(device))
print(y.size())
summary(net, (1, 3, 32, 32), depth=5)
##########################
# Training #
##########################
def train(epoch):
print('nEpoch: %d' % epoch)
net.train()
train_loss = 0
correct = 0
total = 0
for batch_idx, (inputs, targets) in enumerate(tqdm(train_loader)):
inputs, targets = inputs.to(device), targets.to(device)
optimizer.zero_grad()
outputs = net(inputs)
loss = criterion(outputs, targets)
loss.backward()
optimizer.step()
train_loss += loss.item()
_, predicted = outputs.max(1)
total += targets.size(0)
correct += predicted.eq(targets).sum().item()
if batch_idx % 50 == 0:
print('tLoss: %.3f | Acc: %.3f%% (%d/%d)'
% (train_loss / (batch_idx + 1), 100. * correct / total, correct, total))
train_loss = train_loss / len(train_loader)
train_acc = 100. * correct / total
print('n', time.asctime(time.localtime(time.time())))
print(' Epoch: %d | Train_loss: %.3f | Train_acc: %.3f%% n' % (epoch, train_loss, train_acc))
return train_loss, train_acc
def test(epoch):
global best_acc
net.eval()
test_loss = 0
correct = 0
total = 0
with torch.no_grad():
for batch_idx, (inputs, targets) in enumerate(tqdm(test_loader)):
inputs, targets = inputs.to(device), targets.to(device)
outputs = net(inputs)
loss = criterion(outputs, targets)
test_loss += loss.item()
_, predicted = outputs.max(1)
total += targets.size(0)
correct += predicted.eq(targets).sum().item()
if batch_idx % 50 == 0:
print('tLoss: %.3f | Acc: %.3f%% (%d/%d)'
% (test_loss / (batch_idx + 1), 100. * correct / total, correct, total))
test_loss = test_loss / len(test_loader)
test_acc = 100. * correct / total
print('n', time.asctime(time.localtime(time.time())))
print(' Epoch: %d | Test_loss: %.3f | Test_acc: %.3f%% n' % (epoch, test_loss, test_acc))
if test_acc > best_acc:
print('------ Saving model------')
state = {
'net': net.state_dict(),
'acc': test_acc,
'epoch': epoch,
}
if not os.path.isdir('checkpoint'):
os.mkdir('checkpoint')
torch.save(state, './checkpoint/model_%d_%.3f.pth' % (epoch, best_acc))
best_acc = test_acc
return test_loss, test_acc
def save_csv(epoch, save_train_loss, save_train_acc, save_test_loss, save_test_acc):
time = '%s' % datetime.now()
step = 'Step[%d]' % epoch
train_loss = '%f' % save_train_loss
train_acc = '%g' % save_train_acc
test_loss = '%f' % save_test_loss
test_acc = '%g' % save_test_acc
print('------ Saving csv ------')
list = [time, step, train_loss, train_acc, test_loss, test_acc]
data = pd.DataFrame([list])
data.to_csv('./train_acc.csv', mode='a', header=False, index=False)
def draw_acc():
filename = r'./train_acc.csv'
train_data = pd.read_csv(filename)
print(train_data.head())
length = len(train_data['step'])
Epoch = list(range(1, length + 1))
train_loss = train_data['train loss']
train_accuracy = train_data['train accuracy']
test_loss = train_data['test loss']
test_accuracy = train_data['test accuracy']
plt.plot(Epoch, train_loss, 'g-.', label='train loss')
plt.plot(Epoch, train_accuracy, 'r-', label='train accuracy')
plt.plot(Epoch, test_loss, 'b-.', label='test loss')
plt.plot(Epoch, test_accuracy, 'm-', label='test accuracy')
plt.xlabel('Epoch')
plt.ylabel('Loss & Accuracy')
plt.yticks([j for j in range(0, 101, 10)])
plt.title('Epoch -- Loss & Accuracy')
plt.legend(loc='center right', fontsize=8, frameon=False)
plt.show()
if __name__ == '__main__':
model_summary()
if not os.path.exists('../GCN/train_acc.csv'):
df = pd.DataFrame(columns=['time', 'step', 'train loss', 'train accuracy', 'test loss', 'test accuracy'])
df.to_csv('./train_acc.csv', index=False)
print('make csv successful !')
else:
print('csv is exist !')
for epoch in range(start_epoch, end_epoch):
train_loss, train_acc = train(epoch)
test_loss, test_acc = test(epoch)
scheduler.step()
save_csv(epoch, train_loss, train_acc, test_loss, test_acc)
draw_acc()
最后
以上就是积极心情为你收集整理的[ 图像分类 ] 经典网络模型实例—— CIFAR10 + ResNet50 详解与复现???? CIFAR10 + ResNet50 图像分类实例???? CIFAR10 + ResNet50 完整代码的全部内容,希望文章能够帮你解决[ 图像分类 ] 经典网络模型实例—— CIFAR10 + ResNet50 详解与复现???? CIFAR10 + ResNet50 图像分类实例???? CIFAR10 + ResNet50 完整代码所遇到的程序开发问题。
如果觉得靠谱客网站的内容还不错,欢迎将靠谱客网站推荐给程序员好友。
发表评论 取消回复